Institutional-Repository, University of Moratuwa
Welcome to the University of Moratuwa Digital Repository, which houses postgraduate theses and dissertations, research articles presented at conferences by faculties and departments, university-published journal articles and research publications authored by academic staff. This online repository stores, preserves and distributes the University's scholarly work. This service allows University members to share their research with a larger audience.
![]() Research Publications | ![]() Thesis & Dissertation | ![]() E- Books |
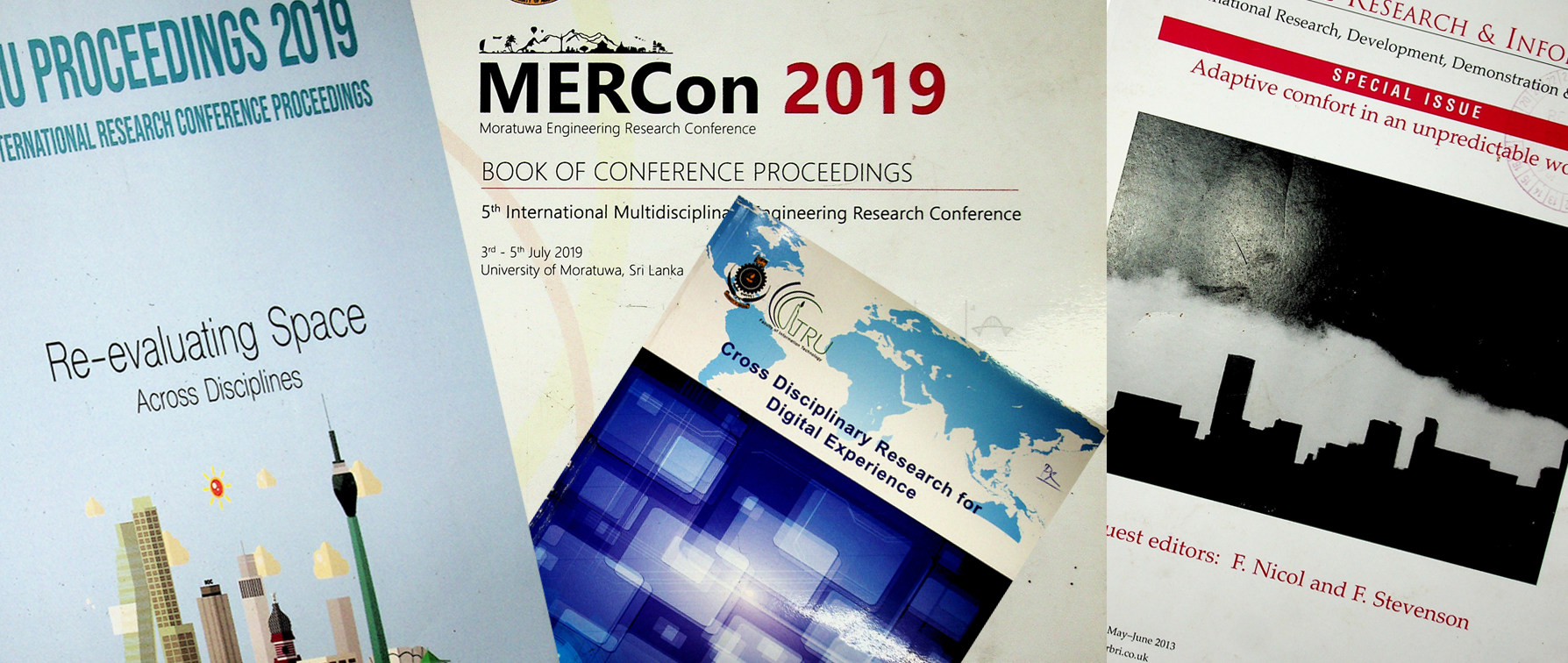
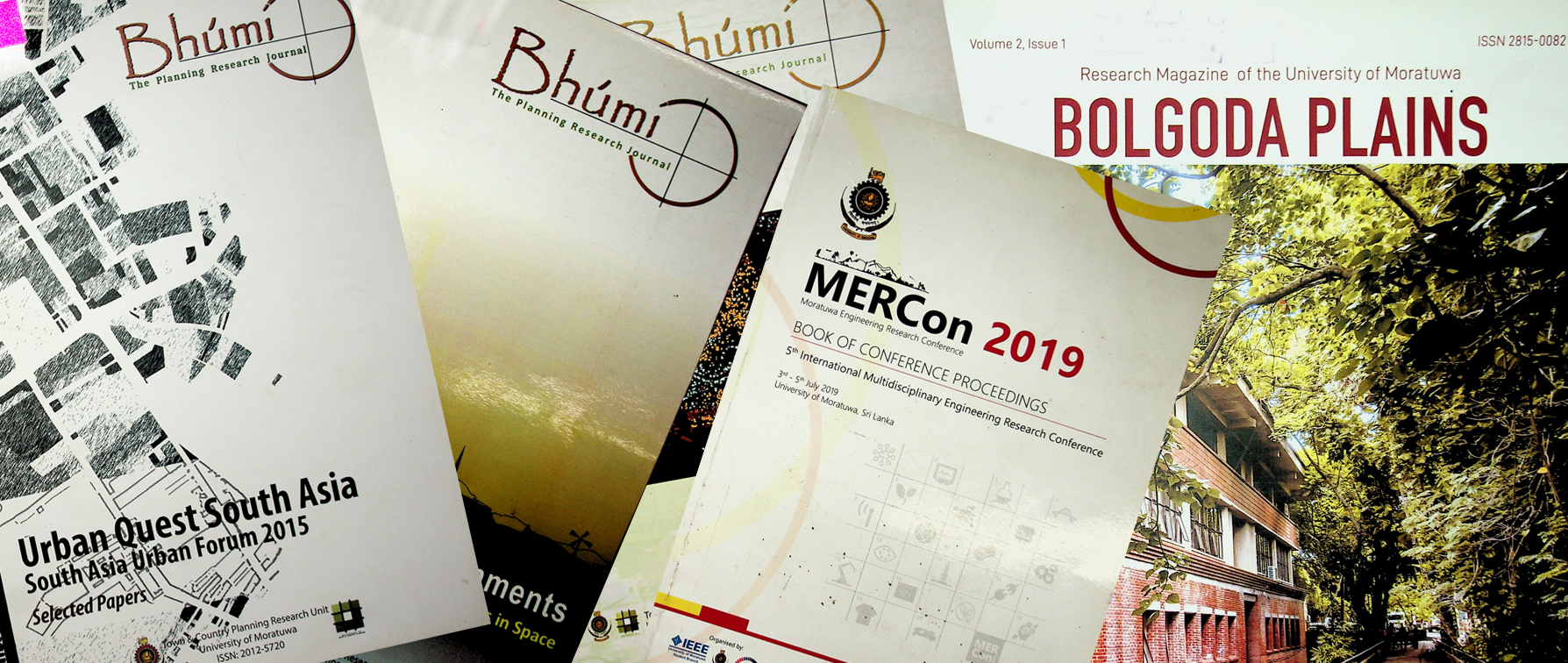
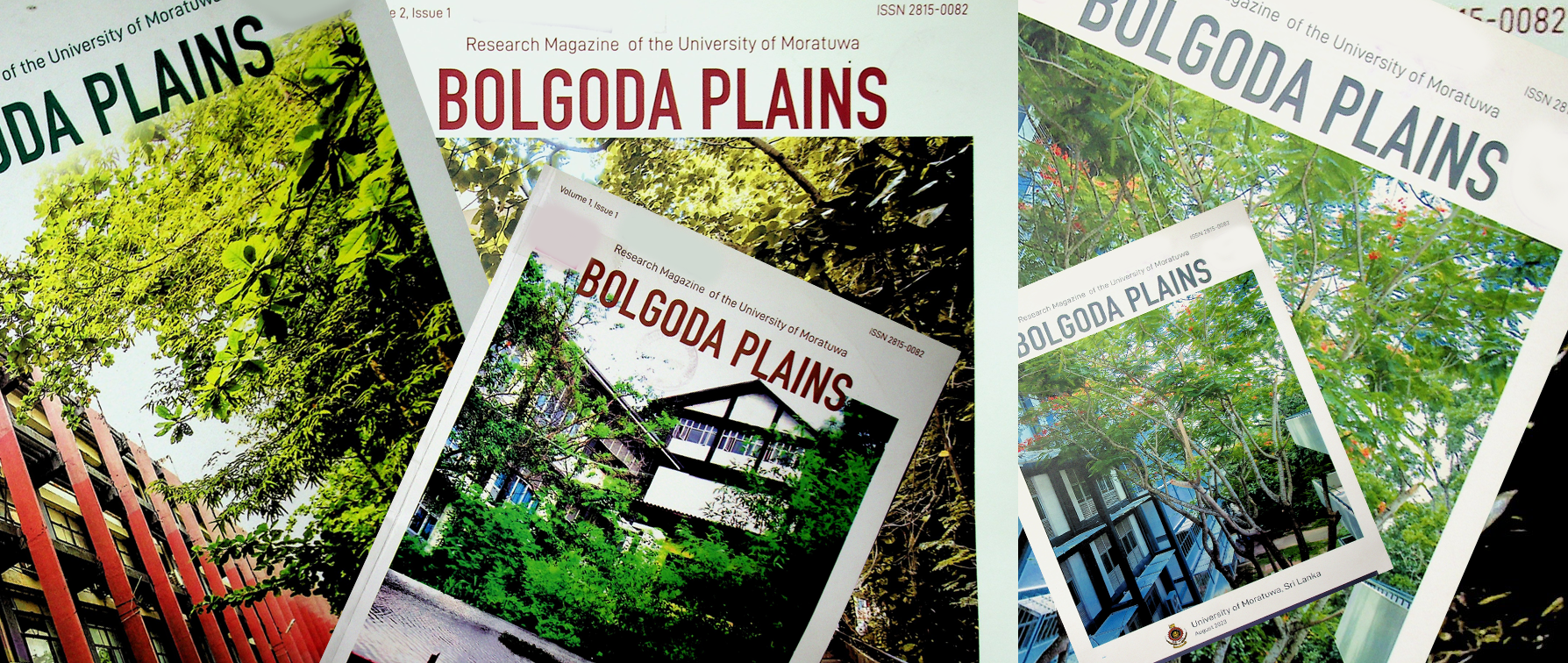
![]() UoM Journal Publications | ![]() UoM Conference Proceedings | ![]() Articles published in Scimago's Q1 journals | ![]() UoM Research Reports | ![]() Other Articles authored by UoM staff |
Recent Submissions
item: Conference-Extended-Abstract
Development and mechanical characterization of particle boards from water hyacinth: a sustainable approach to invasive species utilization
(Engineering Research Unit, 2024) Dharmarathne, GGSBK; Weerasinghe, WDC; Jayalath, DGWT; Priyamantha, JDC; Gamage , JR; Nandasiri , GK; Mawathage , SA; Herath, RP
The worldwide timber consumptions is more than 483 m³ which include the pulp, wood based furniture and goods production which cause a tread for the green environment. It is a material which required and have a high demand for construction, manufacturing, furniture and other packaging item industries. At the same time, this causes series of environmental issues which leads for flooding and global warming. Researchers started to address these issues by giving alternative solutions for usage of wood bio mass with different fiber sources which can be extracted from the waste materials [1]. This study focused on the usage of water hyacinth (Eichhornia crassipes) as an alternative fiber source which can produce particle boards as a sustainable alternative solution for wood based boards. The water hyacinth plants indigenous to Amazon Basin and currently wide spread in several countries including Sri Lanka. It was introduced in 1904 as a free floating decorated plant and spread and went out of control while damaging the eco system of the water reservoirs. It causes deterioration of water quality, minimize the oxygen dissolvement, enhance the cost for upkeeping reservoir and irrigation systems [2], [3]. There are several methods used to control the proliferation of the water hyacinth plant using biological control methods with weevils of the species Neochetina eichhorniae and N. bruchi introduction which were not succeeded as planned [4]. This paths to conduct the research to achieve the alternative solution for wood based products using water hyacinth biomass as a solution for the invasive plants and minimize the ecological issues raised from the deforestation.
item: Conference-Extended-Abstract
Automated data-driven system for natural rubber coagulation using coconut water as a coagulant
(Engineering Research Unit, 2024) Sewwandi, HPGPA; Pallegedara, PGMPB; Varathaseelan, SD; Kumara, IN; Rupasiri,CI; Nanumura, U; Gamage , JR; Nandasiri , GK; Mawathage , SA; Herath, RP
The natural rubber industry covers a wide range of industries, from automotive to healthcare; the efficient and sustainable production of rubber sheets is therefore indispensable. Conventionally, coagulation of rubber involves the use of chemical coagulants like formic acid, which, though effective in their operation, pose serious health risks to workers, including respiratory and skin irritations, especially upon long-term exposure [1]. Besides that, such chemicals contribute to environmental degradation, and shifting toward safer and more sustainable alternatives should be the immediate concern.
Coconut water has emerged as a promising natural coagulant due to its inherent acidity, developed during fermentation, which facilitates the coagulation of rubber. It has been reported that the quality of the rubber sheets produced using coconut water is comparable to those produced using chemical agents [2]. In addition, the use of coconut water reduces the health risks for workers and moves toward the global drive to have environmentally friendly industrial practices.
However, natural coagulants like coconut water have their own challenges in the application process: variations in environmental conditions and the need for precise monitoring generally create a lack of consistency in production. This paper presents a novel automated system with integration of IoT sensors, machine learning algorithms, and blockchain technology to address some of the issues identified above [3]. IoT sensors monitor, in real-time, critical parameters such as pH, temperature, and coagulation time to maintain optimum conditions along the process. Machine learning models using historical and real-time data predict the quality of rubber sheets to enable the detection of anomalies early on and proactively make adjustments. Blockchain further enhances this with an immutable record of production to ensure traceability and consumer trust [4].
item: Conference-Extended-Abstract
Wi-Fi CSI-Based region detection for adaptive video compression and localization
(Engineering Research Unit, 2024) Radhakrishnan, Y; Karki, J; Lenka, MK; Chakraborty, A; Gamage, JR; Nandasiri , GK; Mawathage , SA; Herath, RP
The growing demand for efficient traffic monitoring and surveillance systems, especially in smart cities, requires continuous video recording and transmission for real-time analysis. Static cameras, such as those monitoring roads or public spaces, often capture footage where large portions of the scene remain unchanged, such as the sky, sidewalks, or areas with no movement. Despite this, high-resolution data is continuously streamed to the cloud, consuming excessive bandwidth and energy. With many of these cameras operating on limited power, it is crucial to find ways to reduce the data load while maintaining relevant information for analytics tasks.
Wi-Fi Channel State Information (CSI) based region detection for adaptive video compression helps reduce bandwidth and energy consumption in traffic monitoring systems. By using CSI as a sensing modality, we can predict which parts of a frame contain important motion or activity within the camera’s view. It identifies active regions while compressing static areas, thereby reducing the need to process and transmit the entire frame. This approach conserves bandwidth and extends battery life, making it ideal for low-power cameras while maintaining the quality of essential video data for real-time analysis.
Traditional encoding techniques, such as AVC (H.264) [1] and HEVC (H.265)[2], often struggle to effectively capture the salient features of a video because they lack contextual awareness of the content within each frame. Most Region of Interest (RoI) based compression techniques depend on analyzing video frames to identify regions of activity, utilizing methods such as motion estimation [3], background subtraction [4], and deep learning-based approaches [5]. However, these methods tend to be computationally intensive and require direct frame analysis, which renders them less suitable for resource-constrained or energy-sensitive applications. In contrast, we argue that relying on the video itself for RoI identification not only consumes considerable energy but also requires significant time investment. Instead, we propose utilizing CSI generated by the existing wireless radio in the node as a more efficient alternative for identifying RoIs.
item: Thesis-Abstract
Latency aware location based task offloading algorithm in edge and cloud computing environment
(2023) Moulana, B; Perera, I
Performance-intensive applications are mainly introduced and used in the market after the evolution of 5G and IoT. Most of them still use cloud-based solutions, where only a portion of the application stays on the front end and the rest of the processing occurs in the cloud. Industries such as smart cities, smart homes, and smart grids have limitations in terms of the performance required to completely move on to cloud-based solutions for processing due to their datacenter locations, as distance gives higher latency and poor application performance. Edge computing is the technology to tackle the bottleneck raised by these performance-intensive applications. Due to the resource limitations of the devices at the edge servers, cloud computing is also required to avoid any resource constraints in the worst-case scenario where both cloud and edge computing resources could be collaboratively used. Also, end devices move very often, and their network characteristics change as their location changes. Finding the best task offloading approach based on the end-device location and finding the best possible node to offload the application task was the major objective of this thesis. Existing studies related to task offloading were reviewed and studied along with their existing algorithms used for mobile end-devices for task offloading using iFogSim2. For better performance of mobile end-device applications, a new algorithm was proposed by including a GeoHashing mechanism for faster processing and searching of locations. Then again, it was identified by the simulation that the proposed method completely utilizes the edge nodes first before offloading to the cloud, which gives better performance for the application modules. Also, it was identified that the performance of overall module placements took less time compared to other existing algorithms like random assignments and bottom-up approaches. With the results, it can be stated that the proposed latency-aware task offloading algorithm performs better than other existing algorithms and is a good fit for mobile end-devices, where it could leverage task offloading to the closest node for better performance of the application.
item: Thesis-Abstract
Machine learning based modeling approach to predict delivery promise time for e-commerce
(2023) Fernando, ISS; Ahangama, S
To smoothly function an online retail business, provision of reliable and fast delivery service is essential. A customer's buying decision on an ecommerce platform is discouraged due to erroneous promise timelines defined at the point of order placement. The ecommerce industry is under increasing pressure to create a data-driven framework that can accurately forecast the distribution of delivery time and set precise promise delivery times. By exploiting the relationships between delivery time and relevant predictor variables, developed tree based model, quantile regression, quantile regression forest model, LightGBM trained with quantile regression model, and LSTM deep learning model to generate conditional distribution forecasts. The models are evaluated using two methods of scoring rules namely pinball loss function and continuous ranked probability score (CRPS). LSTM model incorporated with quantile regression generated best results out of all developed, trained and tested from real world dataset obtained from ABC.com - Sri Lanka’s largest ecommerce platform, where multi-origin marketplace seller fulfillment was considered as the novelty contribution. Furthermore, it was revealed that hour of order placement and origin to destination combination impacted most on delivery time and highlighted the importance of integrating the forecasting model to decision making structure. As a limitation of this study and eligible future work, forecasting the delivery time with most recent order distribution restricting to the ongoing logistics capacity utilizations can be stated.And also consideration on incorporating real-time data and cost-sensitive approaches to improve the practicality and effectiveness of forecasting in e-commerce businesses